On December 6, 2022, the series of One Hundred International Scholars Seminar Report from the Faculty of Computing was held online. At the invitation of Associate Professor Liu Bingquan from Faculty of Computing, Dr. Arkaitz Zubiaga from Queen Mary University of London brought an academic report with the theme of Generalization in Social Media Research: from Fact Verification to Hate Speech Detection to teachers and students.
Associate Professor Arkaitz Zubiaga is the director of the Social Data Science Laboratory at Queen Mary University in London. His research direction is social data science. His research interest is to connect online data with events in the real world, and to solve problems on the Internet and social media that may have a devastating impact on individuals or the whole society, such as hate speech, false information, inequality, prejudice and other forms of online harm.
Dr. Arkaitz Zubiaga has published more than 130 papers (including more than 50 journal papers) and been cited more than 5300 times (h index 36). He served as the academic editor of six journals, and was also a (S) PC member of several top international conferences in the fields of computational social sciences (WWW, ICWSM, CHI, CSCW), natural language processing (ACL, EMNLP, COLING), and artificial intelligence (IJCAI, AAAI, WSDM).
In this academic report, Dr. Arkaitz Zubiaga, starting from the necessity and challenges of model generalization, introduced in detail the model generalization methods of fact verification and hate speech detection tasks based on social media data for different model generalization scenarios. Dr. Arkaitz Zubiaga focused on sharing a series of work carried out by his team in the social media research direction in recent years, and introduced how to improve the ability of models for fact verification and hate speech detection tasks in cross language generalization, cross platform generalization and cross time generalization. In the final question and answer session, Dr. Arkaitz Zubiaga patiently answered questions for teachers and students about model bias, cross language social media analysis, and topic selection for doctoral students in the context of big model and big computing power.
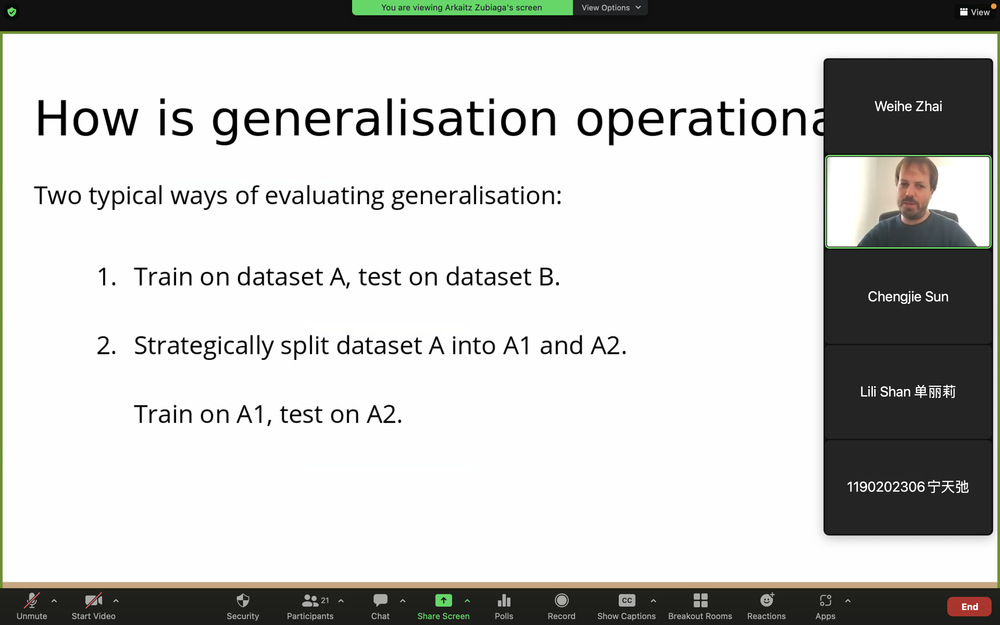
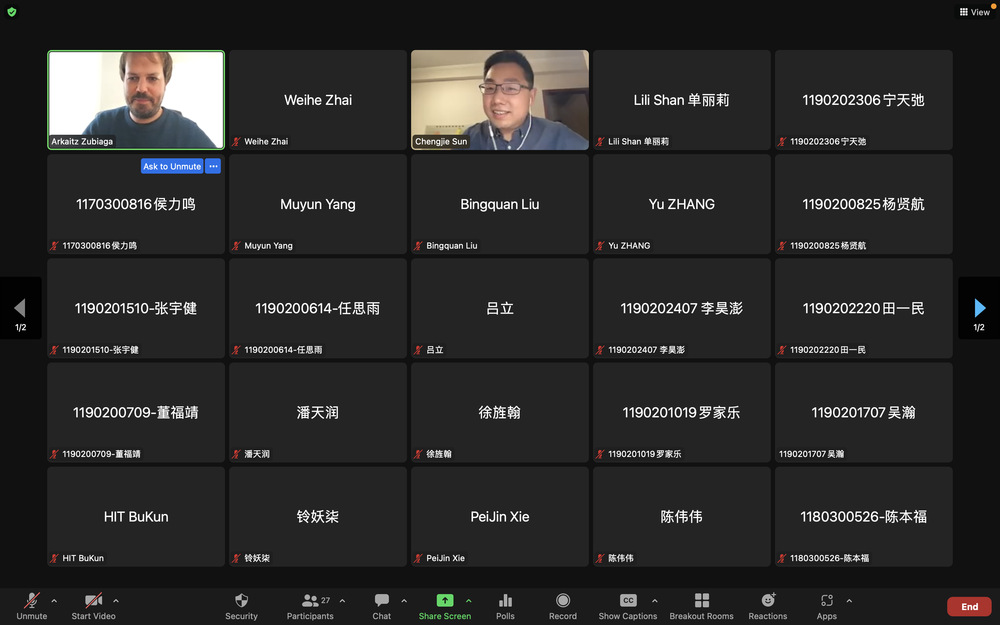